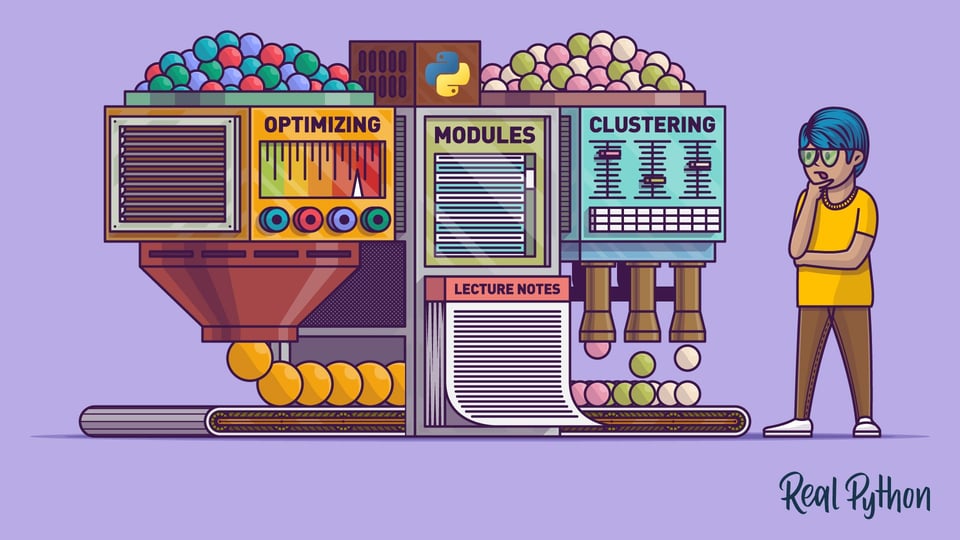
The other day, while working on fpdf2,
I used @dataclass
,
a nice decorator that came in the standard library with Python 3.7,
to quickly define a class
that mostly stored data.
Then a question came to my mind: is the __slots__
memory optimization compatible with @dataclass
?
Is it even compatible?.
This very short article is basically an opportunity to answer those questions with some minimal code, mostly as a reminder to myself:
#!/usr/bin/env python
import os, sys
from collections import namedtuple
from dataclasses import dataclass
from typing import NamedTuple
def get_process_rss(): # Similar to: psutil.Process().memory_info().rss / 1024 / 1024
try:
with open(f"/proc/{os.getpid()}/statm", encoding="utf8") as statm:
rss_as_mib = int(statm.readline().split()[1]) * os.sysconf("SC_PAGE_SIZE") / 1024 / 1024
return f"{rss_as_mib:.1f} MiB"
except FileNotFoundError: # /proc files only exist under Linux
return "<unavailable>"
class Object:
def __init__(self, x, y, z):
self.x = x
self.y = y
self.z = z
class ObjectWithSlots:
__slots__ = ('x', 'y', 'z')
def __init__(self, x, y, z):
self.x = x
self.y = y
self.z = z
@dataclass
class Dataclass:
x: int
y: int
z: int
@dataclass(slots=True) # since Python 3.10, this is the same as defining __slots__ manually
class DataclassWithSlots:
x: int
y: int
z: int
Namedtuple = namedtuple('E', ('x', 'y', 'z'))
class NamedTupleSubclass(NamedTuple):
x: int
y: int
z: int
def Tuple(x, y, z):
return (x, y, z)
def Dict(x, y, z):
return locals()
Class = locals()[sys.argv[1].upper()]
l = []
for _ in range(100000):
l.append(Class(0, 1, 2))
print(get_process_rss())
Results on my machine with Python 3.8:
$ ./slots_test.py Object
26.6 MiB
$ ./slots_test.py ObjectWithSlots
17.2 MiB
$ ./slots_test.py Dataclass
26.7 MiB
$ ./slots_test.py DataclassWithSlots
17.2 MiB
$ ./slots_test.py Namedtuple
19.1 MiB
$ ./slots_test.py NamedTupleSubclass
19.2 MiB
$ ./slots_test.py Tuple
17.8 MiB
$ ./slots_test.py Dict
34.5 MiB
Results on my machine with Python 3.10 in debug mode:
$ ./slots_test.py Object
28.3 MiB
$ ./slots_test.py ObjectWithSlots
22.0 MiB
$ ./slots_test.py Dataclass
28.3 MiB
$ ./slots_test.py DataclassWithSlots
22.0 MiB
$ ./slots_test.py Namedtuple
24.8 MiB
$ ./slots_test.py NamedTupleSubclass
24.2 MiB
$ ./slots_test.py Tuple
24.3 MiB
$ ./slots_test.py Dict
38.3 MiB
We can conclude that:
__slots__
is still an effective memory optimization with recent versions of Python, that can provide between 10% en 30% of RAM savings__slots__
can effectively be combined with@dataclass
namedtuple
/NamedTuple
is less memory-efficient than__slots__
(and both cannot be combined)
Related content:
- Official Python documentation on
__slots__
- Blog article @py.checkio.org
- Les slots, une optimisation méconnue, a Frenc blog article pointing that using
__slots__
also makes code faster - StackOverflow post on Python
__slots__
Well aware of the limitations of __slots__
, I will definitely adopt more of them in fpdf2
😊
Since december, I have been working on tracking memory allocations occuring during the execution of fpdf2
unit tests suite,
and this is no easy task: issue #641.
I stil haven't found what I'm looking for, the main difficulty being the opacity of the Python memory allocator,
and tracking the memory allocated through malloc
calls by libraries that fpdf2
depends on,
but it has been very insightful so far! 😁